DeepMind, Google’s AI research and development lab, believes that advancing AI systems could involve tackling complex geometry problems. The lab recently introduced AlphaGeometry, a system claiming to solve as many geometry problems as an average International Mathematical Olympiad gold medalist. This open-source code outperforms its predecessors, solving 25 Olympiad geometry problems within the standard time limit compared to the previous state-of-the-art system’s 10.
The Significance of Olympiad-Level Geometry
DeepMind considers solving Olympiad-level geometry problems a crucial milestone in developing deep mathematical reasoning, aiming for more advanced and general AI systems. Google AI research scientists Trieu Trinh and Thang Luong emphasize in a blog post that this achievement could open new possibilities across mathematics, science, and AI.
The Role of Geometry in AI Advancement
DeepMind argues that proving mathematical theorems requires both reasoning and the ability to choose from various steps toward a solution. This problem-solving approach could potentially contribute to the development of more capable and general-purpose AI systems in the future.
Unique Challenges in Training AI for Geometry
Training an AI system to solve geometry problems presents unique challenges due to the complexities of translating proofs into machine-understandable formats. The scarcity of usable geometry training data and the limitations of current generative AI models further complicate the process.
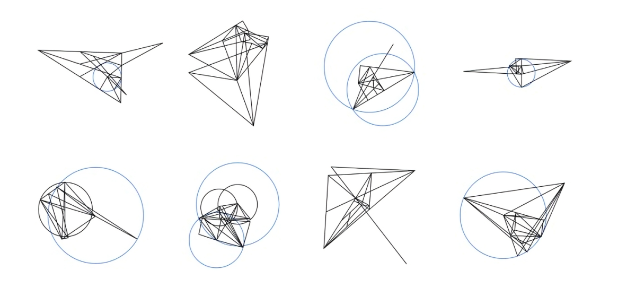
AlphaGeometry’s Design Approach
DeepMind addressed these challenges by pairing a “neural language” model, similar to ChatGPT, with a “symbolic deduction engine.” This hybrid system utilizes synthetic data generated by the lab, consisting of 100 million synthetic theorems and proofs of varying complexity. The neural model guides the deduction engine through possible answers to geometry problems, providing both intuitive ideas and deliberate, rational decision-making.
Symbolic vs. Neural Network Approaches
The results of AlphaGeometry‘s problem-solving, published in a study in the journal Nature, fuel the ongoing debate between symbolic and neural network approaches in AI. While neural networks like AlphaGeometry have shown success in various tasks, proponents of symbolic AI argue for the efficiency of encoding knowledge, reasoning through complex scenarios, and explaining decision-making processes.
A Hybrid Path Forward
AlphaGeometry, as a hybrid symbolic-neural network system, raises the possibility that combining both approaches could be the optimal path toward generalizable AI. Similar to DeepMind’s successes with AlphaFold 2 and AlphaGo, AlphaGeometry exemplifies the potential synergy between symbol manipulation and neural networks in the quest for advanced AI.
Long-Term Goals: Generalizable AI Systems
DeepMind’s long-term goal is to build AI systems capable of generalizing across mathematical fields and exhibiting sophisticated problem-solving and reasoning abilities. The aim is to extend the frontiers of human knowledge, shaping how future AI systems discover new knowledge in mathematics and beyond.